A study published on arXiv highlights Apple’s assessment of various leading language models, including those from OpenAI, Meta, and other major developers, focusing on their ability to perform mathematical reasoning tasks.
The results show that even minor changes in how questions are phrased can lead to significant variations in the models’ performance, raising concerns about their reliability in tasks requiring logical consistency.
Apple underscores a persistent issue with language models: their dependence on pattern matching rather than true logical reasoning.
In several tests, the researchers illustrated how adding irrelevant information to a question—details that should not impact the mathematical result—caused the models to produce drastically different answers.
One example from the study involved a basic math question asking how many kiwis a person gathered over a series of days.
When irrelevant details, such as the size of some of the kiwis, were introduced, models like OpenAI’s o1 and Meta’s Llama incorrectly modified the final total, despite these details having no relevance to the solution.
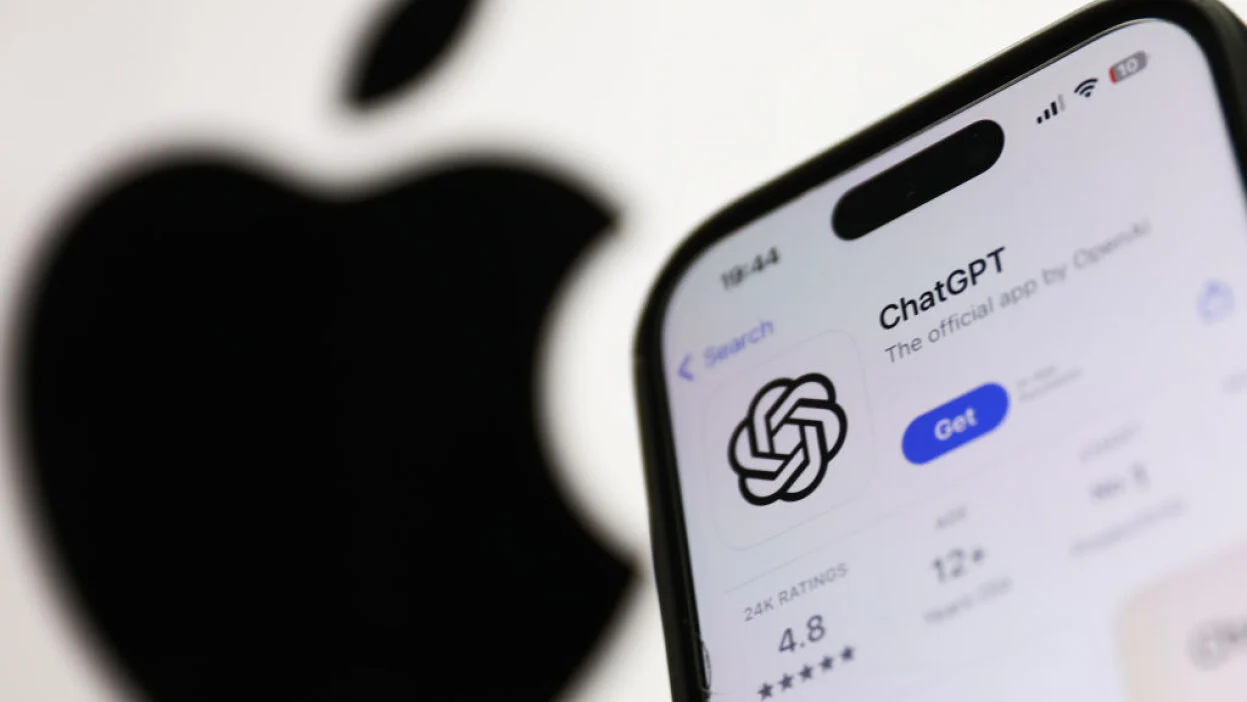
The researchers noted:
“We found no evidence of formal reasoning in language models. Their behavior is better explained by sophisticated pattern matching—so fragile, in fact, that changing names can alter results by ~10%.”
This fragility in reasoning led the researchers to conclude that language models do not use real logic to solve problems but instead rely on complex pattern recognition learned during their training.
They discovered that “simply changing names can alter results,” which raises concerns about the future of AI in applications requiring consistent and reliable reasoning in real-world situations.
According to the study, all tested models, ranging from smaller open-source models like Llama to proprietary ones like OpenAI’s GPT-4o, experienced significant performance drops when presented with seemingly insignificant variations in the input data.
Apple suggests that in order to achieve more accurate decision-making and problem-solving abilities, AI might need to combine neural networks with traditional, symbol-based reasoning, a concept known as neurosymbolic AI.