By now, anyone following advancements in AI research is likely familiar with generative models that create speech or melodic music from simple text prompts.
Nvidia’s recently revealed “Fugatto” model takes this concept further, introducing innovative training techniques and inference-level combination methods to “transform any mix of music, voices, and sounds” while synthesizing entirely new sounds that have never existed.
Though Fugatto isn’t publicly available yet, a demo-packed website showcases its impressive capabilities.
Users can adjust distinct audio traits and descriptions, leading to creations ranging from saxophones that bark to voices speaking underwater or ambulance sirens forming a harmonious choir.
While the results vary in quality, the breadth of possibilities highlights Nvidia’s assertion that Fugatto is “a Swiss Army knife for sound.”
The Importance of High-Quality Data
In a research paper, Nvidia explains the challenge of building a dataset capable of revealing meaningful connections between audio and language.
Unlike standard language models, which often derive insights from text-based data, audio models require more explicit guidance to interpret and replicate specific traits or descriptions.
To address this, the researchers used a large language model (LLM) to generate Python scripts that create diverse instructions for describing audio “personas” (e.g., “standard, young-crowd, thirty-somethings, professional”).
These instructions included both absolute prompts (e.g., “synthesize a happy voice”) and relative prompts (e.g., “increase the happiness of this voice”) to refine the model’s ability to modify traits.
Most open-source audio datasets lack embedded trait annotations, so the team employed existing audio analysis models to generate “synthetic captions” for training clips.
These captions provided natural language descriptions to quantify elements like emotion, gender, and speech quality.
Additional audio processing tools analyzed acoustic traits, such as “reverb” or “fundamental frequency variance,” ensuring a robust dataset.
For relational comparisons, datasets featuring controlled variables—such as emotional variations of the same sentence or different instruments playing identical notes—helped the model understand how specific traits manifest.
By processing these and other open-source collections, the team developed a heavily annotated dataset with over 20 million samples spanning 50,000 hours of audio.
Training Fugatto on this data with Nvidia tensor cores yielded a model boasting 2.5 billion parameters, achieving consistent results across various audio quality metrics.
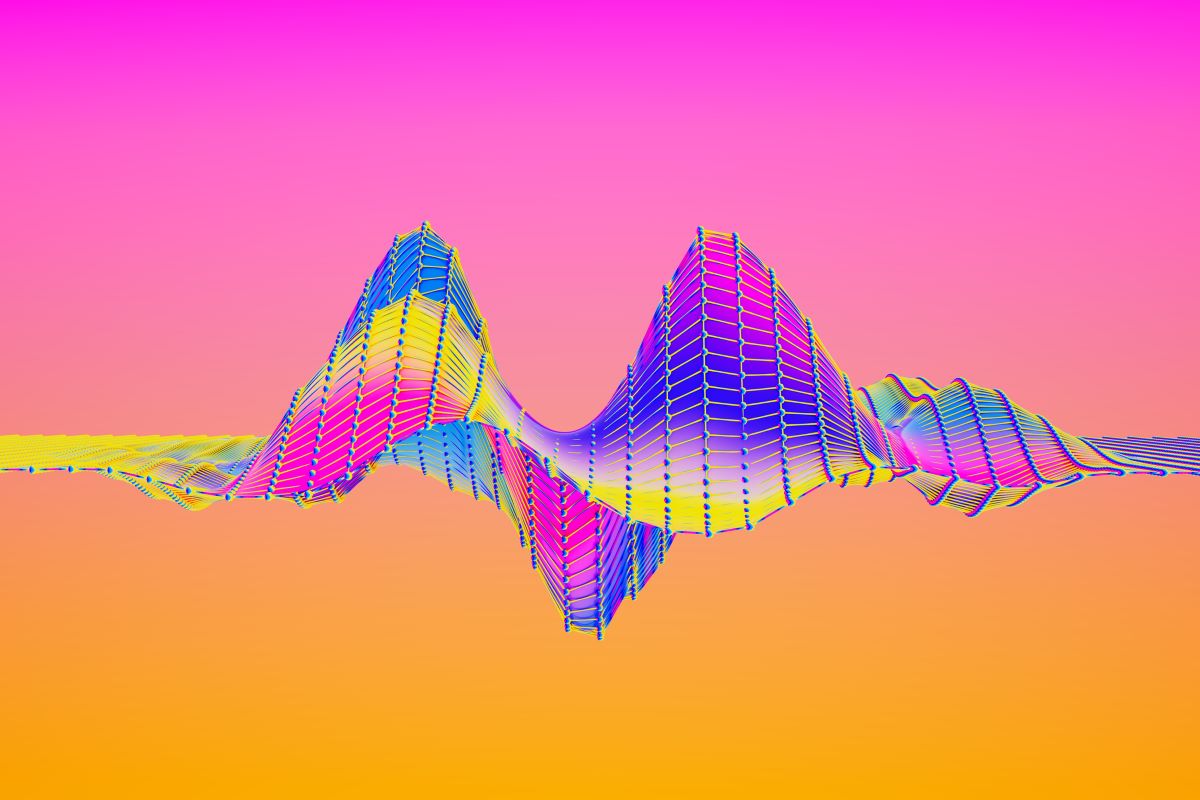
Transformative Audio Mixing with ComposableART
Nvidia has highlighted Fugatto’s “ComposableART” system (short for “Audio Representation Transformation”) as a cornerstone of the model’s capabilities.
This feature enables Fugatto to process prompts—including text, audio, or both—using “conditional guidance” to independently control and generate unique combinations of audio instructions.
This means Fugatto can synthesize sounds that fall outside its training data, creating entirely new auditory experiences.
For example, ComposableART allows Fugatto to produce a violin that “sounds like a laughing baby,” a banjo playing under gentle rainfall, or factory machinery emitting metallic screams.
While some generated sounds are more convincing than others, the ability to merge disparate elements demonstrates how Fugatto can interpret and combine traits across datasets.
A standout feature is Fugatto’s treatment of audio traits as a tunable continuum rather than binary values.
For instance, blending the sounds of an acoustic guitar and running water produces significantly different results depending on how the model weights each element.
Similarly, Fugatto can adjust the strength of a French accent or the “degree of sorrow” in spoken dialogue.
Beyond its unique synthesis capabilities, Fugatto performs tasks common to prior models, such as altering the emotion in speech, isolating vocals from music, or modifying beats in a track by adding synchronized effects like barking dogs or ticking clocks.
It can also replace individual notes in MIDI files with a variety of vocal performances or other sounds, aligning perfectly with the input melody.
Potential Applications and Artistic Value
While Fugatto is an early step “towards a future where unsupervised multitask learning emerges from data and model scale,” Nvidia envisions diverse applications for the model.
These include song prototyping, dynamically adjusting video game scores, and tailoring audio for international advertising campaigns.
However, Nvidia emphasizes that Fugatto is a tool for enhancing artistic creativity rather than replacing it.
In an Nvidia blog post, music producer and songwriter Ido Zmishlany reflected on AI’s role in the evolution of music:
“The history of music is also a history of technology. The electric guitar gave the world rock and roll. When the sampler showed up, hip-hop was born.
With AI, we’re writing the next chapter of music. We have a new instrument, a new tool for making music—and that’s super exciting.”
Whether creating unprecedented sounds or augmenting traditional audio workflows, Fugatto stands as a testament to the transformative potential of AI in music and sound design.
Its ability to blend and manipulate audio traits not only pushes technical boundaries but also offers new avenues for artistic exploration.